Maximizing Operational Efficiency: Leveraging IoT Integration, Data Visualization, and Predictive Maintenance for Process Stability and Optimal Performance
- satyashukla
- Mar 5
- 3 min read
In an era where technological advancements are rapidly changing the landscape of manufacturing and production, operational monitoring has become a cornerstone of achieving maximum efficiency. The integration of Internet of Things (IoT) devices, robust data visualization tools, and predictive maintenance techniques are at the heart of this transformation. This blog post delves into how these elements work together to enhance process stability and ensure optimal performance.
IoT Integration: Real-Time Data Collection
The integration of IoT devices into machinery and production lines heralds a new age of operational monitoring. By deploying sensors and connected devices, companies can now collect real-time performance data from various aspects of their operations. This advancement enables managers and operators to obtain insights into machine performance, production output, and any anomalies that may arise during the manufacturing process.
With real-time data, teams are equipped to make informed decisions dynamically, rather than relying on historical data alone. This immediate feedback loop assists in promptly addressing issues before they escalate into larger problems. As such, IoT integration not only improves response times but also enhances overall efficiency in manufacturing processes.
Data Visualization: Tracking Performance Metrics
Once data is collected through IoT devices, the next step is to leverage cutting-edge data visualization tools. These tools are vital in transforming complex data sets into comprehensible visual forms, such as graphs and dashboards. By tracking key performance metrics over time, companies can easily identify trends and detect anomalies that may require attention.
Effective data visualization enhances transparency and collaboration among team members. When everyone has access to the same performance metrics, it fosters a culture of proactive problem-solving and continuous improvement. Moreover, visualizing data helps communicate findings more clearly to stakeholders who may not be familiar with technical jargon but need to understand operational performance.
Process Stability: Ensuring Consistency
Control Charts: The Monitoring Standard
To maintain process stability, control charts play a crucial role in monitoring manufacturing processes. These statistical tools enable companies to visualize variations in production processes, thereby ensuring that operations remain stable and within predefined control limits. By consistently tracking these metrics, businesses can identify any deviations that warrant corrective actions.
Control charts provide a framework for understanding process behavior and implementing necessary adjustments. As a result, manufacturers can reduce variability and maintain quality standards, ultimately leading to more consistent product output and enhanced customer satisfaction.
Statistical Analysis: Identifying Variations
The use of statistical analysis techniques is fundamental in understanding the root causes of variations in manufacturing processes. By applying methods such as hypothesis testing, regression analysis, and variance analysis, companies can dissect the factors influencing performance metrics.
Through these analyses, organizations are better positioned to identify which variables significantly impact efficiency and quality. This insight allows for timely corrective actions that can mitigate or eliminate unwanted variations, paving the way for sustained operational performance.
Predictive Maintenance: A Proactive Approach
Historical Data Analysis: Anticipating Failures
One of the most significant advantages of integrating IoT into manufacturing is the ability to conduct historical data analysis for predictive maintenance. By examining past performance data, organizations can forecast when machinery is likely to require maintenance before a failure occurs. This proactive approach significantly reduces unexpected downtime, thereby optimizing the overall production schedule.
Understanding failure patterns through historical data empowers companies to implement maintenance strategies that align with their operational goals. By minimizing disruptions caused by equipment failures, manufacturers can enhance productivity and reduce maintenance costs.
Machine Learning: Enhancing Predictive Models
To further refine predictive maintenance efforts, machine learning algorithms are employed to analyze both historical and real-time data. These algorithms can recognize patterns that humans might overlook, leading to more accurate predictions regarding equipment failures.
Machine learning models continuously improve as more data is collected, allowing for enhanced forecasting accuracy over time. Consequently, organizations can schedule maintenance at the optimal time, ensuring that machinery operates smoothly without the surprises of unplanned maintenance.
Conclusion
In conclusion, maximizing operational efficiency requires a multifaceted approach that combines IoT integration, data visualization, and predictive maintenance strategies. By leveraging these technologies, companies can enhance process stability, maintain consistent performance, and ultimately achieve their manufacturing goals.
The landscape of manufacturing is evolving, and businesses that adopt these innovative techniques will not only stay ahead of the competition but also set new standards for operational excellence. Embracing IoT, effective data analysis, and predictive maintenance is no longer an option but a necessity for organizations aiming to thrive in the 21st-century production environment.
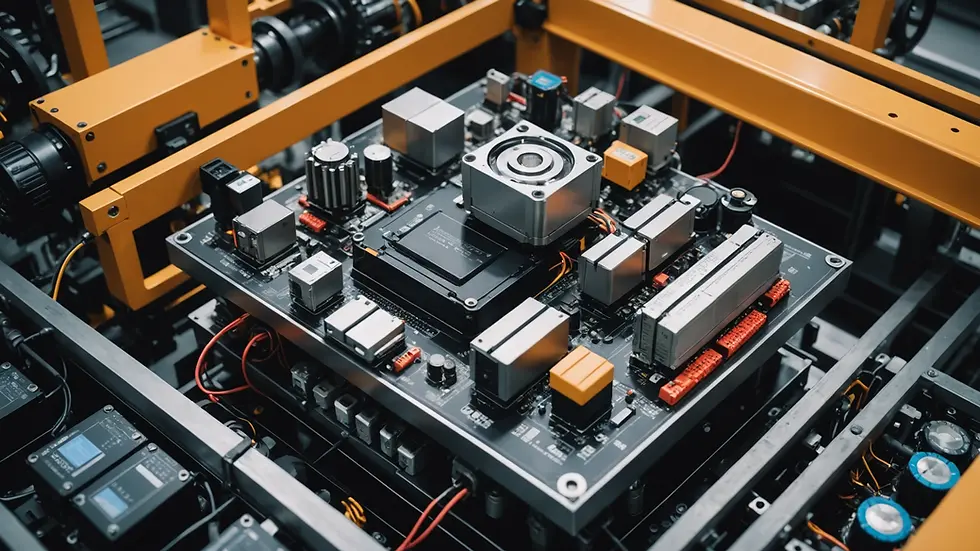
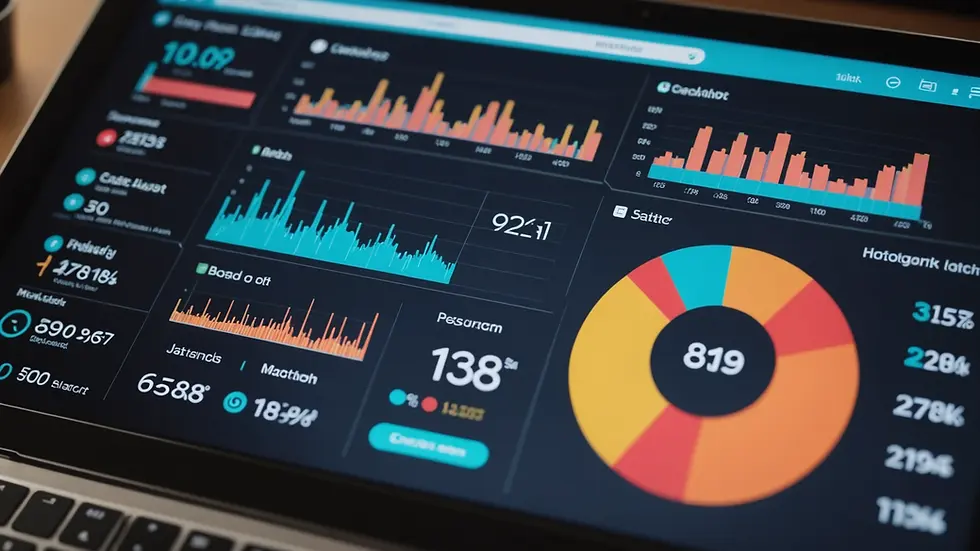
Comments